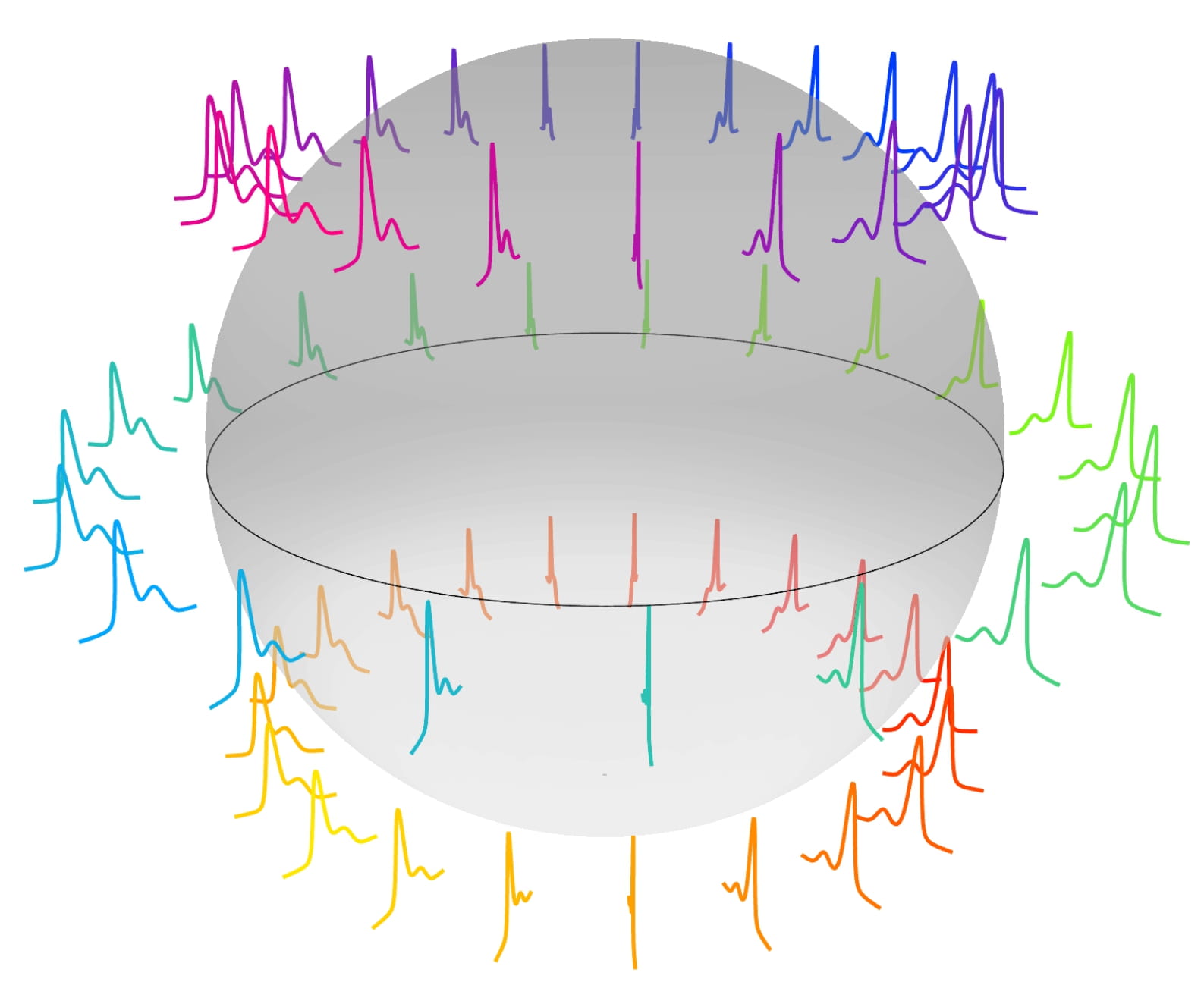
Small Transformers Compute Universal Metric Embeddings
A Kratsios, V Debarnot, I Dokmanić, 2022
A. Kratsios, V. Debarnot, and I. Dokmanić: Small Transformers Compute Universal Metric Embeddings, JMLR - Journal of Machine Learning Research, 2023.
S. Hou, P. Kassraie, A. Kratsios, A. Krause, and J. Rothfus: Instance-dependent generalization bounds via optimal transport, JMLR - Journal of Machine Learning Research, 2023.
A. Kratsios and L. Papon: Universal Approximation Theorems for Differentiable Geometric Deep Learning, JMLR - Journal of Machine Learning Research, 2022.
Acciaio, B., Kratsios, A., and Pammer, G., Designing Universal Causal Deep Learning Models: The Geometric (Hyper) Transformer, Mathematical Finance - Special Issue on Machine Learning in Finance
A. Kratsios and C. Hyndman: NEU: A Meta-Algorithm for Universal UAP-Invariant Feature Representation, JMLR - Journal of Machine Learning Research, 2021.
G. Alvarez, I. Ekren, A. Kratsios, X. Yang: Neural Operators Can Play Dynamic Stackelberg Games, 2024.
T. Furuya, A. Kratsios: Simultaneously Solving FBSDEs with Neural Operators of Logarithmic Depth, Constant Width, and Sub-Linear Rank, 2024.
R. Hong and A. Kratsios: Bridging the gap between approximation and learning via optimal approximation by ReLU MLPs of maximal regularity, 2024.
Focus: Mathematical and statistical foundations of (geometric deep and operator) learning. Applications of Interest: Deep learning for optimal control, game theory, PDEs, and finance.
A Kratsios, V Debarnot, I Dokmanić, 2022
A. Accaio, A. Kratsios and G. Pammer, Mathematical Finance, 2022.
A. Kratsios and B. Zamanlooy, TMLR, 2022.
R. Hong and A. Kratsios
R. Saquer, Y. Limmer, A. Kratsios, F. Krach, B. Horvath, etc, ICLR, 2025.
H. Borde Ocariz, A. Kratsios, T. Law, X. Dong, M. Bronstein, ICLR, 2025.
T. Cheng, A. Lucci, A. Kratsios, D. Belius, NeurIPS, 2024.
M. Persiianov, A. Asadulaev, N. Andreev, A. Kratsios, A. Korotin, E. Burnaev, etc
P. Casgrain and A. Kratsios, COLT, 2021.
L Galimberti, G Livieri, A Kratsios, 2022.
A. Kratsios and C. Hyndman, JMLR, 2021.
A. Kratsios, B. Zamanlooy, I. Dokmanic, T. Liu, ICLR (Spotlight), 2022
A. Kratsios and B. Zamanlooy, Neurocomputing, 2022.
S Hou, P Kassraie, A Kratsios, J Rothfuss, A Krause, 2022.
A. Kratsios, 2021.
LC Herrera, F Krach, A Kratsios, P Ruyssen, J Teichmann, 2021.
I host a organize and host a weekly AI theory seminar, powered by: the Vector Institute, exploring the most recent results in AI theory. Our seminar covers topics ranging from statistical and approximation-theoretic guarantees to the theoretical viability of deep learning solutions to problems in stochastic analysis and game theory.
Past and present group members.
Postdoctoral Researcher (2022-2025) - AI in Game Theory and Stochastics
PhD Candidate (2023-2027) - Foundations of AI
PhD Candidate (2022-2026) - Deep Clustering
MSc to PhD Candidate (2024-2028) - Foundations of Geometric Deep Learning
PhD Candidate (2025-2029) - AI Reasoning (Joint: Bradd Hart)
MSc Candidate (2022-2024) - Operator Learning in Finance
Co-Supervised at: Scuola Normale Superiore di Pisa
MSc (2023-2025) - Hypernetworks for time-series prediction (CSE)
NSERC BSc Math (2024-2028) - Statistical Learning Theory
BSc Math 2024, Summer Researcher - Adaptive Gradient Descent
Computer Engineering 2025, Summer Researcher - Deep Learning
Honors BSc Math (2024-2025) - Foundations of Geometric Deep Learning